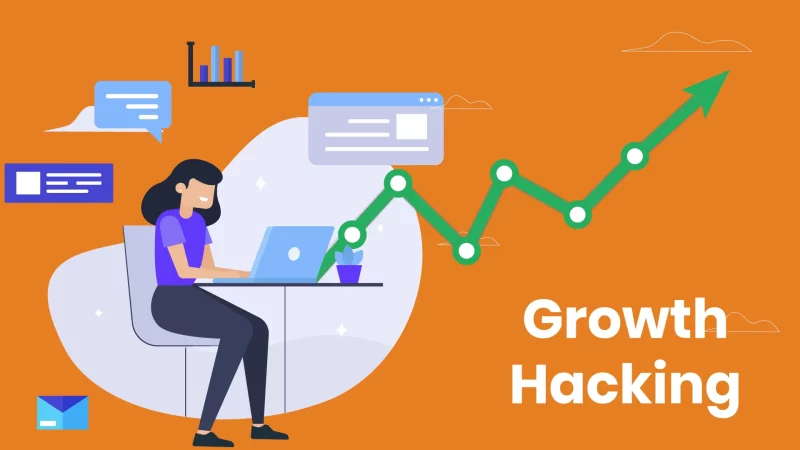
In addition, for readers who are looking to learn more about Growth Hacking, this article can also serve as an overview of the processes and skills required to work in a position related to Growth Hacking. .
STEP 1: BUILDING DATA APPLICATION ENVIRONMENT
The biggest problem a company starting out with Growth Hacking is often the lack of a data collection and processing environment that can be analyzed for Growth Hacking.
Usually, Startup companies can build a Growth Team with main tasks such as:
- Select and manage key metrics.
- Design and build data pipelines.
- Analyze data by subject.
- Respond to export requests and analyze data from other parties.
- Build a working environment based on data-based culture.
However, for some startups that lack human resources, having a fully qualified Growth team to perform all of the above tasks is also quite difficult, but fortunately we live in an era with a lot of IaaS, PaaS , SaaS for data analysis development. By using solutions available from these third parties such as cloud analytics platforms, ETL automation solutions, Business Intelligence solutions, there is no need for too many resources and capabilities. Engineering can also build a data-driven environment that underpins Growth Hacking.
Cloud Analytics Platform (Cloud Analytics Platform):
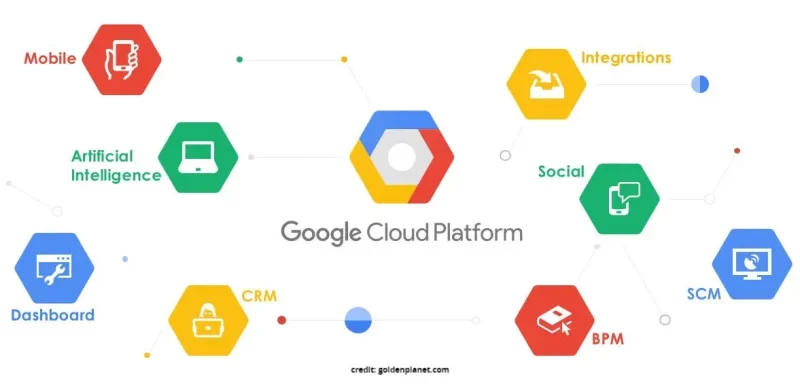
By using Cloud Analytics solutions, steps like data collection, storage and pre-processing are simplified and made much easier with just a few steps of installation. Some typical cloud computing platforms (Cloud Platform) can be mentioned as Amazon’s EMR, Google’s GCP, Microsoft Azure, ..
ETL Automation Solution:
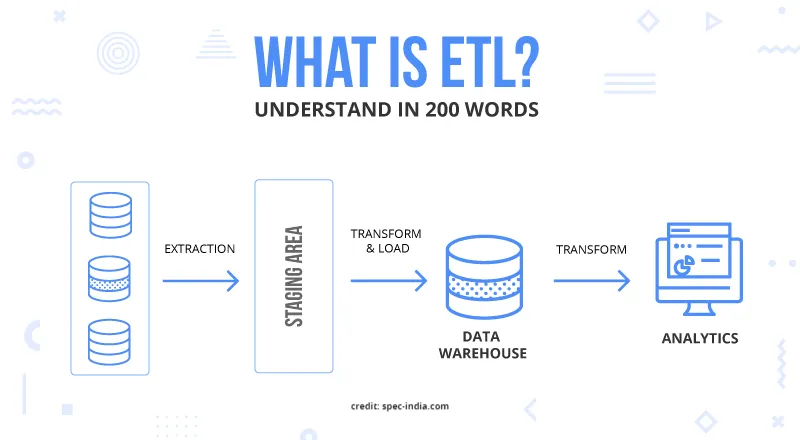
After collecting and storing, before you can conduct data analysis, it is necessary to perform the ETL process (Extract – extract, Transform – transform, Load – load). ETL is the process of collecting data from multiple sources, processing this data source into a form that is easier to analyze, and finally loading the data into the target database. The reason for taking this step is because the data generated during the operation of the service is different in form and condition from the data that can be used for analysis.
ETL automation solutions like Fivetran, Stitch load into the final database normalized data by chaining dozens of different data sources such as advertising platforms, Attribution, CRM, …
Business Intelligence (BI) Solution:
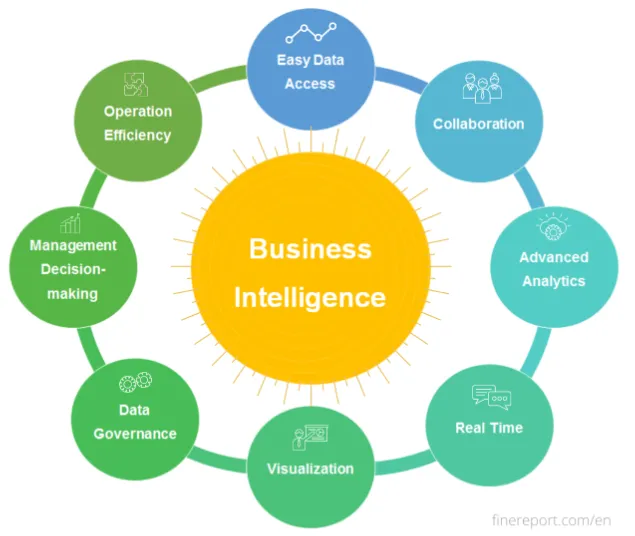
Since Growth Hacking requires the coordination of multiple positions with different technical abilities (Product Development and Marketing), the data environment for Growth Hacking also needs to be friendly to non-technical positions. data analysis techniques. The data aggregation and visualization dashboard is a tool that ensures every location is easily accessible and gathers insights from the data.
Currently, solutions like Tableau or Google Data Studio are very developed, so positions without a background in analysis or development can still use it easily.
STEP 2: BUILD THE DATA PIPLINE
From the above solutions, it is possible to build a basic data pipeline without too many technical resources. Data pipeline is the entire process from data collection, data processing, data export, and data use to arrive at a data-driven decision to serve the needs of the business.
In addition, to build a data pipeline, it is extremely important to decide what kind of data to measure. Data exists in the form of logs. There are two types of logs to distinguish: the service log and the action log.
- Service log is responsible for recording transaction results. When a transaction such as membership registration, reservation or payment is successful, a service log is recorded.
- Action log records each user action (action) on the service such as clicking on products, searching, adding to cart.
Service log is the basic data that must be managed to run the service. Therefore, when starting Growth Hacking, companies usually do not have many problems with the collection and application of this data. In contrast, with action logs, the amount of data is many times larger and the design process has more variables, making it relatively more difficult to collect and apply. A company when starting a Growth Hack needs to pay attention to this issue.
STEP 3: BUILD CULTURE AND CAPACITY FOR DATA APPLICATION
In any working environment, the human element is indispensable. After building the basic environment for data adoption, we need to focus on building the right culture and capacity to take advantage of this environment.
At the most basic level, (depending on the organization of each company) each individual position related to Growth Hack tasks or a member of the Growth Hack team needs to be able to use the company’s BI tools and understand how to read and build dashboards that serve their needs.
A little more in-depth, knowledge of data extraction and processing with SQL is also quite useful because the most recently used BI tools have support for querying and processing data using SQL.
STEP 4: GROWTH TEST: TEST A/B
- Definition : A/B testing is the process of testing two different options, to see which is more effective based on the results of measuring user response.
- Hypothesis : must clearly define the goal, the direction you want to test through experiment. Proceed by identifying the independent and dependent variables and then decide the target of the dependent variable. For example: “In the service introduction screen, the conversion rate will be higher if the service is presented with a picture than with a textual service”.
- Independent Variable : Also known as the explanatory or predictive variable. In a causal relationship, it can be seen as causal, that is, the variable is expected to affect the dependent variable. An independent variable is any change to one of the design elements, each layout of a website or email to test to improve conversion rates or other KPIs. For example, in the above hypothesis, “form of presentation of “product introduction”” will be the independent variable.
- Dependent Variable : The variation that is expected to be influenced by the independent variable. In a causal relationship, it can be seen as the result. While testing, we will measure many criteria, but in each test should only choose and focus on one criterion. This criterion is the dependent variable. For example, in the above hypothesis, “swap rate” is the dependent variable.
- Control Variable (Control Variable) : This is not an independent variable but a variable 3, which can affect the dependent variable. For example, in the above hypothesis, one of the typical controlling variables is Funnels (paid ads, friend referral, ..)
- Sample size : This is the number of people participating in the experiment to test the hypothesis. This amount shall be determined before the test is carried out and shall be large enough to show a difference between the two samples. Currently, there are many online tools to help find the right sample size for each A/B experiment, just enter the design conditions of the A/B experiment such as hypothesis, testability, and criteria to make the experiment. becomes meaningful, etc., then these tools will calculate for you the sample size. Some typical sites include https://www.evanmiller.org/ab-testing/ or https://docs.adobe.com/content/target-microsite/testcalculator.html
- Testing time : After deciding on the sample size, we have to decide how long it will take to collect enough data for hypothesis testing.
Points to keep in mind when doing A/B testing:
- No hypothesis
- Failure to manage control variables
- In addition to the values representing the central trend (mean, median, mode), it is necessary to consider the data comprehensively: distribution, significance level, effect size ) etc
- Do not observe, compare changes over time
- Too much trust in past A/B Test experience: Results can change at any time with various reasons such as changes in the market, changes in user groups, etc.
Epilogue:
The key point of Growth Hacking is data, but for Growth Hacking to be successful, quantity of data is not as important as quality. This quality is the result of both technology and people. A good Growth Hacker or Growth Hacking team will build a logical path to get that useful data. Hopefully, some of the above suggestions will help you somewhat on the way to conquering Growth Hacking.